Empowering Businesses with Innovative Solutions
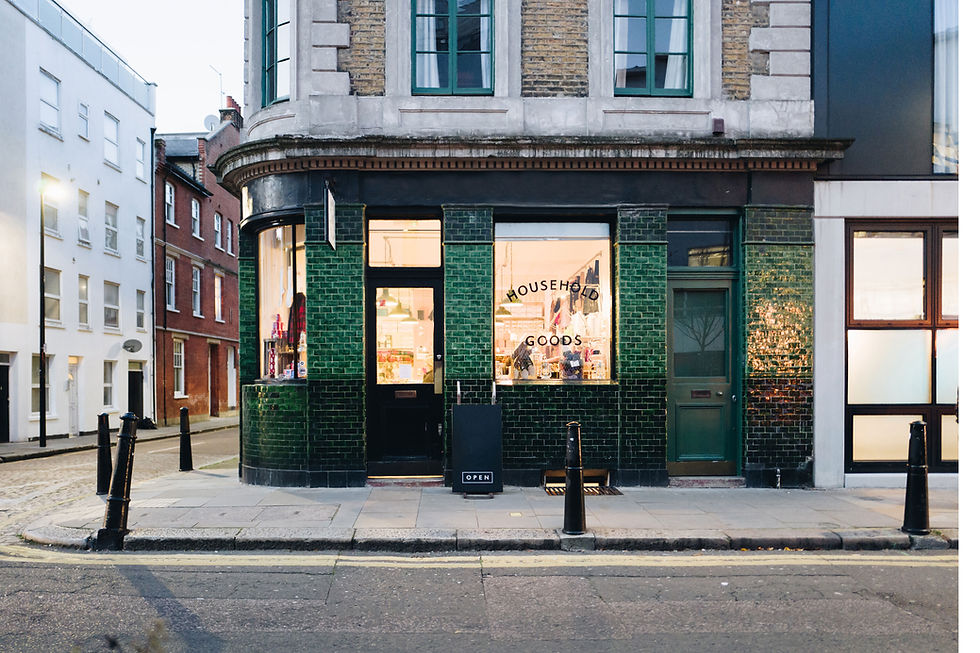
Case Study: Walmart – AI-Powered Demand Forecasting for Operational Excellence
Optimize inventory management and reduce stockouts/overstocking across thousands of stores while accounting for demand variability caused by seasonality, economic factors, and localized events.
Case Study: Walmart – AI-Powered Demand Forecasting for Operational Excellence
Overview of the Business
Company: WalmartIndustry: RetailEmployees: ~2.1 million globallyBusiness Challenge: Optimize inventory management and reduce stockouts/overstocking across thousands of stores while accounting for demand variability caused by seasonality, economic factors, and localized events.
Problem Statement
Walmart operates on razor-thin margins in a highly competitive retail landscape. The key challenges include:
Inventory Inefficiencies: Overstocking leads to high storage costs and waste (e.g., perishables), while stockouts result in lost sales and dissatisfied customers.
Demand Volatility: Seasonal peaks, unpredictable trends, and local buying patterns make demand forecasting complex.
Scale of Operations: Managing inventory across 10,000+ stores requires advanced, automated tools that can provide accurate forecasts in real-time.
Strategic Solution: AI-Powered Demand Forecasting
To address these challenges, Walmart implemented AI-driven demand forecasting to improve inventory management, reduce operational costs, and enhance customer satisfaction. By leveraging machine learning algorithms and real-time data integration, Walmart was able to align inventory with customer demand accurately.
Key Features Implemented
Data-Driven Demand Forecasting
Walmart deployed advanced machine learning models (e.g., time series, regression, and neural networks) to predict product demand across stores.
Data Inputs:
Historical sales data from POS systems.
Real-time trends (e.g., weather, holidays, local events).
Macroeconomic indicators and external market data.
Customizable Forecast Models
AI models were tailored for product categories (e.g., perishables, apparel, electronics).
Specific variables like weather data influenced forecasts for items like umbrellas and winter clothing.
Scenario Planning
Walmart simulated “what-if” scenarios to adjust inventory decisions:
Promotional impact forecasting.
Price elasticity testing to assess demand sensitivity.
Real-Time Data Integration
Integrated real-time sales and supply chain data into their automated replenishment systems.
Enabled immediate adjustments to forecasts based on actual market signals.
Visualization and Reporting
Walmart used custom dashboards to visualize forecast accuracy and inventory levels, making it easier for store managers to act on insights.
Implementation Breakdown
Phase 1: Discovery and Data Audit
Walmart conducted an extensive audit of its historical sales and supply chain data:
Key Insight: 20% of stockouts occurred during localized weather events that weren’t accounted for in prior forecasts.
Phase 2: Model Selection and Customization
Walmart leveraged Google Cloud AI and custom models using Python-based tools (TensorFlow, ARIMA, and Prophet).
Models were trained to:
Recognize seasonal patterns.
Incorporate external data streams like weather APIs and local event calendars.
Phase 3: Deployment
Walmart rolled out automated demand forecasting tools integrated into their replenishment systems.
Forecasts were generated at store-level granularity for over 100,000 SKUs.
Phase 4: Training and Alignment
Store managers and supply chain teams were trained on interpreting and acting upon forecast recommendations.
Phase 5: Ongoing Support and Optimization
AI models were continuously retrained with new data for improved accuracy.
Regular performance reviews identified gaps and opportunities for further optimization.
Results
Reduced Stockouts and Overstocking
Stockouts: Reduced by 30%, leading to increased sales and improved customer satisfaction.
Overstock Costs: Decreased by 20%, significantly reducing inventory holding expenses.
Improved Forecast Accuracy
Forecast accuracy improved to 90%+ for key product categories, enhancing decision-making at scale.
Operational Efficiency
Automated inventory replenishment allowed Walmart to minimize manual intervention.
Faster response to demand surges reduced supply chain bottlenecks.
Cost Savings and Revenue Growth
Walmart reported annual savings of $1 billion through optimized inventory management.
Transferability to Small and Medium-Sized Businesses (SMBs)
Walmart’s success with AI-powered demand forecasting demonstrates principles and tools that SMBs can adopt:
Affordable Tools
Platforms like AWS Forecast, Google Cloud AI, or Microsoft Azure ML offer pre-built models tailored for SMBs.
Smaller businesses can use tools like QuickBooks Commerce, Zoho Analytics, or Tableau for demand visualization.
Demand Forecasting Implementation
Data Sources: SMBs can start with sales data from POS systems and incorporate external factors like local events.
Forecast Models: Use accessible time series tools like Prophet or Excel-based models for initial demand predictions.
Scenario Planning
SMBs can simulate “what-if” scenarios for promotions or seasonal demand spikes to align inventory and resources.
Cost Savings
Avoiding stockouts or overstocking can lead to 10–20% cost savings on inventory.
Small retailers and manufacturers can reduce waste and improve cash flow.
Example SMB Use Case: Local Grocery Store Chain
Client: A regional grocery chain with 15 stores.Problem: Frequent stockouts of seasonal products (e.g., fresh produce) and overstock of slow-moving items.
Solution:
Implemented AI demand forecasting tools to analyze POS sales and local weather trends.
Automated inventory replenishment based on predicted demand spikes.
Results:
Reduced stockouts by 40%.
Decreased perishable food waste by 25%.
Increased customer satisfaction and repeat visits by 15%.
Conclusion
Walmart’s AI-powered demand forecasting showcases how businesses can leverage data-driven insights to optimize inventory, reduce costs, and improve customer satisfaction. For small and medium-sized businesses, similar tools can be implemented at a fraction of the cost, offering significant operational improvements and competitive advantages.
By adopting AI-powered demand forecasting, SMBs can ensure they anticipate customer needs, operate efficiently, and drive sustainable growth.
Power in Numbers
30
Programs
50
Locations
200
Volunteers