Empowering Businesses with Innovative Solutions
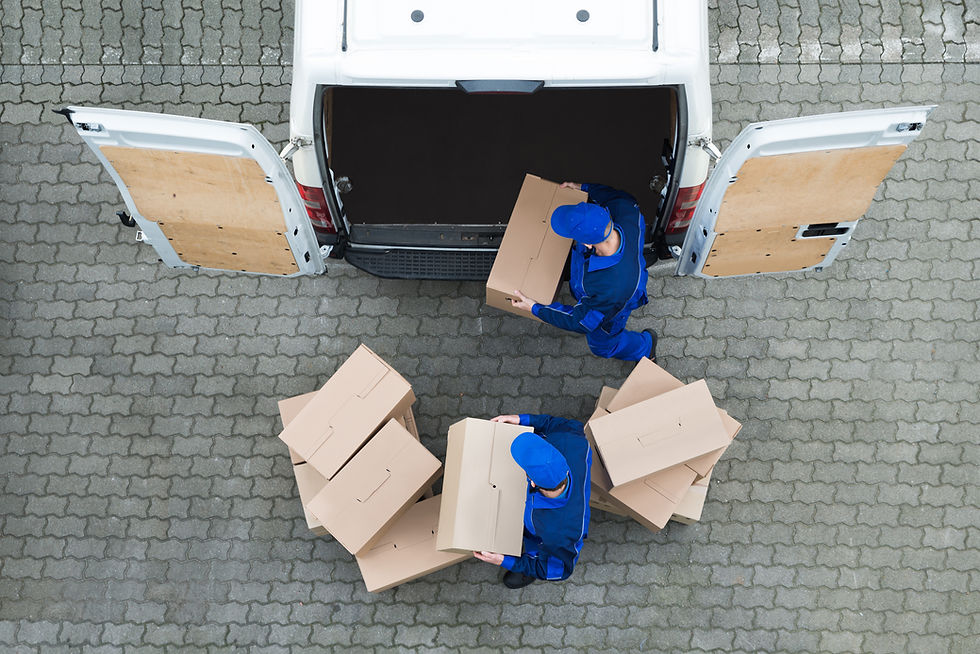
Case Study: Workforce Scheduling Optimization at United Parcel Service (UPS)
Optimizing workforce scheduling for dynamic delivery demand, reducing operational costs, ensuring employee satisfaction, and maintaining regulatory compliance.
Case Study: Workforce Scheduling Optimization at United Parcel Service (UPS)
Overview of the Business
Company: United Parcel Service (UPS)Industry: Logistics and Supply ChainEmployees: ~500,000 globallyBusiness Challenge: Optimizing workforce scheduling for dynamic delivery demand, reducing operational costs, ensuring employee satisfaction, and maintaining regulatory compliance.
Problem Statement
UPS faces unique workforce scheduling challenges due to:
Variable Demand: Demand fluctuates heavily based on daily, weekly, and seasonal delivery volumes, especially during holidays and e-commerce spikes.
Labor Costs: Rising overtime expenses and inefficiencies due to suboptimal workforce management.
Employee Burnout: Unequal shift distribution led to high fatigue levels and dissatisfaction among drivers.
Operational Complexity: Managing a global workforce across time zones, legal compliance, and diverse logistical demands.
Strategic Solution: Smart Workforce Scheduling
To solve these challenges, UPS implemented AI-driven workforce scheduling using a combination of demand-based planning tools and optimization algorithms. The solution streamlined the scheduling process, reduced inefficiencies, and improved employee satisfaction.
Key Features Implemented
Demand-Based Scheduling
Tool: AI algorithms integrated with UPS’s ORION (On-Road Integrated Optimization and Navigation) platform.
Approach:
Used historical data (past delivery trends, traffic patterns, weather data).
Forecasted workforce demand at specific times and locations.
Scheduled drivers dynamically to match delivery peaks and troughs.
Employee Preference Integration
Incorporated driver preferences and availability via a mobile app.
Allowed for shift swaps and flexible scheduling.
Compliance and Fairness
Embedded local labor laws (overtime limits, mandated rest hours) into the scheduling algorithms.
Ensured equal distribution of work hours to prevent overburdening staff.
Real-Time Adjustments
Integrated real-time traffic and demand spikes into scheduling, dynamically adjusting driver assignments.
Example: A last-minute demand surge in e-commerce deliveries triggered automated workforce redeployment.
Mobile Access for Employees
Provided drivers with access to schedules via mobile apps.
Allowed seamless communication and visibility of changes.
Analytics and Reporting
Used dashboards to track workforce productivity, overtime trends, and satisfaction levels.
Implementation Breakdown
Phase 1: Discovery and Needs Assessment
UPS conducted a workforce analysis to pinpoint:
Peak demand hours (e.g., during holidays like Black Friday).
Labor cost trends and inefficiencies.
Driver dissatisfaction due to schedule conflicts.
Key Finding: Up to 30% of driver shifts had either overstaffing or understaffing issues during peak delivery times.
Phase 2: Tool Selection and System Design
Selected AI scheduling tools integrated with ORION and third-party systems like Workforce.com.
Developed optimization models using machine learning and operations research algorithms (e.g., OR-Tools by Google).
Phase 3: Pilot and Deployment
Tested the system in selected regional hubs for one month.
Gathered data and employee feedback to fine-tune algorithms.
Phase 4: Training and Rollout
Conducted workshops for managers and employees.
Launched a mobile app for drivers to monitor and manage schedules.
Phase 5: Ongoing Support and Optimization
Monitored KPIs: overtime costs, employee satisfaction, and delivery efficiency.
Continued refining AI models based on new data.
Results
Labor Cost Reduction
Overtime Reduced: By 15% in the first six months, saving an estimated $100 million annually.
Improved alignment between workforce demand and delivery volume.
Employee Satisfaction
Flexible scheduling reduced driver fatigue and increased job satisfaction scores by 20%.
Real-time communication via mobile apps improved transparency.
Operational Efficiency
Delivery Productivity: Optimized workforce scheduling improved on-time deliveries by 12%.
Real-time adjustments allowed UPS to meet demand spikes seamlessly without overstaffing.
Regulatory Compliance
Reduced non-compliance incidents (e.g., exceeding overtime limits) by 80%.
Transferability to Small and Medium-Sized Businesses
While UPS operates on a global scale, the principles and tools used in this case can be applied to small and medium-sized businesses (SMBs):
Demand-Based Scheduling
Tools for SMBs: Platforms like Deputy, Shiftboard, or When I Work.
SMBs can forecast workforce needs using historical sales or production data.
Employee Preference Management
Mobile-access scheduling tools allow employees to report availability and request changes seamlessly.
Cost Optimization
Reduce unnecessary overtime and labor costs by aligning workforce schedules with demand.
Real-Time Adjustments
Small businesses in logistics, retail, or manufacturing can quickly redeploy staff to handle demand fluctuations.
Compliance Automation
Labor laws and compliance rules can be embedded in tools like Deputy or Shiftboard to ensure legal adherence.
Scalability
SMBs can start with simple off-the-shelf scheduling tools and later integrate advanced AI features as they grow.
Example SMB Application: Regional Retail Chain
Business: A regional retail chain with 10 stores and 150 employees.
Problem: High labor costs and low employee morale due to inconsistent scheduling.
Solution: Implemented Deputy for demand-based scheduling.
Results:
Reduced overtime by 25% annually.
Improved employee satisfaction by 30% through fairer and flexible schedules.
Saved $50,000 in labor costs.
Conclusion
The UPS case study highlights the immense value of AI-driven workforce scheduling, demonstrating significant cost savings, improved operational efficiency, and higher employee satisfaction. These strategies, powered by accessible tools, can be scaled down and implemented by small and medium-sized businesses to optimize their operations, drive growth, and ensure sustainable success.
Power in Numbers
30
Programs
50
Locations
200
Volunteers